A New Dataset and Benchmark for Content-aware Visual-Textual Presentation Layout
Welcome to the official webpage of PKU PosterLayout!
It is a dataset and benchmark for content-aware visual-textual presentation layout. We first introduced it in our CVPR 2023 paper.
Properties and Specialties:
As described in our paper, we define three types of elements (i.e., text, logo, and underlay).
Examples of poster-layout pairs and image canvases are shown in Figure 1 and 2.
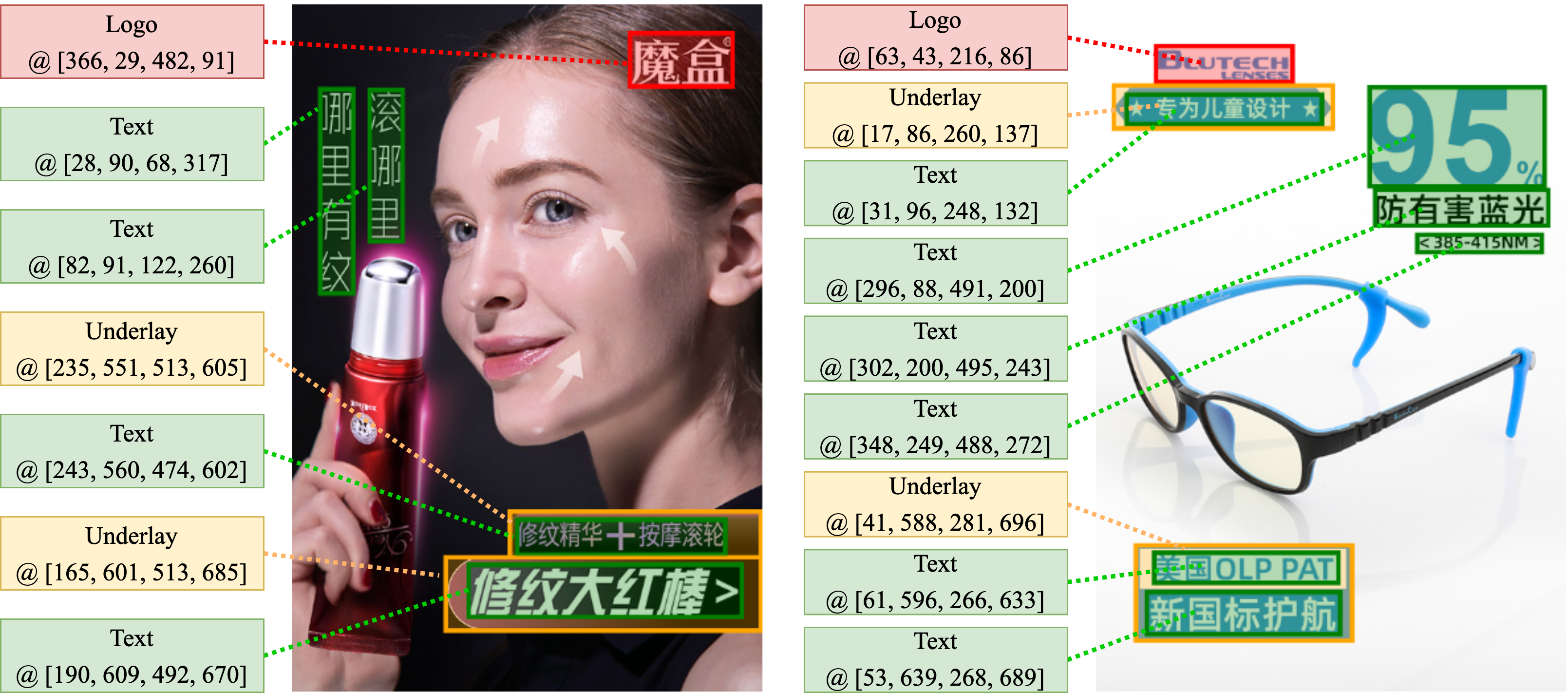
Figure 1. Examples of poster-layout pairs.
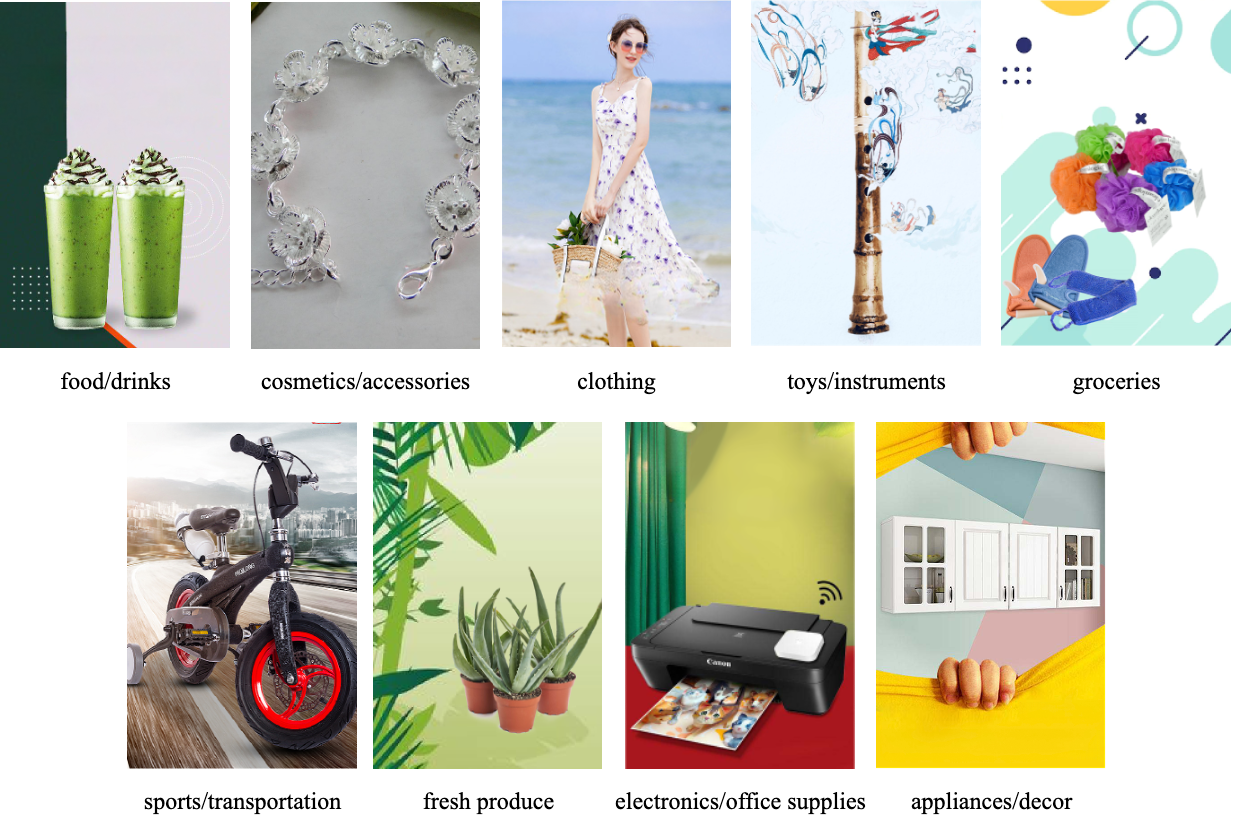
Figure 2. Examples of image canvases.
It provides challenges and traits on three aspects:
- Domain diversity
We collected data from multiple sources, including an e-commerce posters dataset [1] and multiple image bank websites [2]. Images are diverse in domain, quality, and resolution, causing shifts in data distributions and making our dataset more general.
- Content diversity
We defined nine categories covering most products, including food/drinks, cosmetics/accessories, electronics/office supplies, toys/instruments, clothing, sports/transportation, groceries, appliances/decor, and fresh produce. Afterward, we collected or built data evenly distributed among these categories, ensuring content diversity.
- Layout variety and complexity
We worked with a professional labeling team consisting of six people to complete the layout annotation work. To avoid annotation errors, we triple-checked the annotations before putting the dataset into use. Moreover, we carefully processed to retain plenty of complex layouts with more than 10 elements.
As the first public dataset containing complex layouts, it provides more difficulties in modeling the intra-layout relationship and stands for an extended task requiring complex layouts.
The composition is shown as follows:
Table 1: Split and data type.
Set |
Data type |
Amount |
Training |
Poster-layout pair |
9,974 |
Testing |
Image canvas |
905 |
All usage of the data must give a citation to the following paper: HsiaoYuan Hsu, Xiangteng He, Yuxin Peng, Hao Kong and Qing Zhang, "PosterLayout: A New Benchmark and Approach for Content-Aware Visual-Textual Presentation Layout", 36th IEEE Conference on Computer Vision and Pattern Recognition (CVPR), Vancouver, Canada, Jun. 18-22, 2023.
Dataset Download:
Images in PKU PosterLayout are distributed under the CC BY-SA 4.0 license. For easier utilization, we provide:
- Original posters
- Inpainted posters, processed using the Fourier-convolution-based inpainting method [3]
- Image canvases
- Saliency maps, generated using PFPN [4] and BASNet [5], of both posters and canvases
Overall, there are 42,611 images with a total size of 7.37 GB. They are accessible via PKU Netdisk(pw: CdXe) and Google Drive.
As for the layout annotations, please sign the Release Agreement and send it to SiBo Yin (2401112164@stu.pku.edu.cn).
By sending the application, you are agreeing and acknowledging that you have read and understand the notice.
We will reply with the file and the corresponding guidelines right after we receive your request!
Experimental Results:
We conducted the canvas content-aware visual-textual presentation layout task and defined eight evaluation metrics, including 5 graphic metrics and 3 content-aware metrics, shown as follows:
(note:↑indicates the higher is the better, ↓indicates the lower is the better)
- Graphic metrics
- Val↑: Element area compliance rate
- Ove↓: Element overlap rate
- Ali↓: Element non-alignment level
- Undl↑: Underlay avaliable rate (loose)
- Unds↑: Underlay avaliable rate (strict)
- Content-aware metrics
- Uti↑: Non-salient area utilization rate
- Occ↓: Salient object occlusion rate
- Rea↓: Text's unreadability level
Here is the leaderboard:
Table 2: Comparison of quantitative results.
Method |
Val ↑ |
Ove ↓ |
Ali ↓ |
Undl ↑ |
Unds ↑ |
Uti ↑ |
Occ ↓ |
Rea ↓ |
SmartText [5] |
- |
- |
- |
- |
- |
0.0849 |
0.0912 |
0.1528 |
CGL-GAN [6] |
0.7066 |
0.0605 |
0.0062 |
0.8624 |
0.4043 |
0.2257 |
0.1546 |
0.1715 |
DS-GAN (Ours) [7] |
0.8788 |
0.0220 |
0.0046 |
0.8315 |
0.4320 |
0.2541 |
0.2088 |
0.1874 |
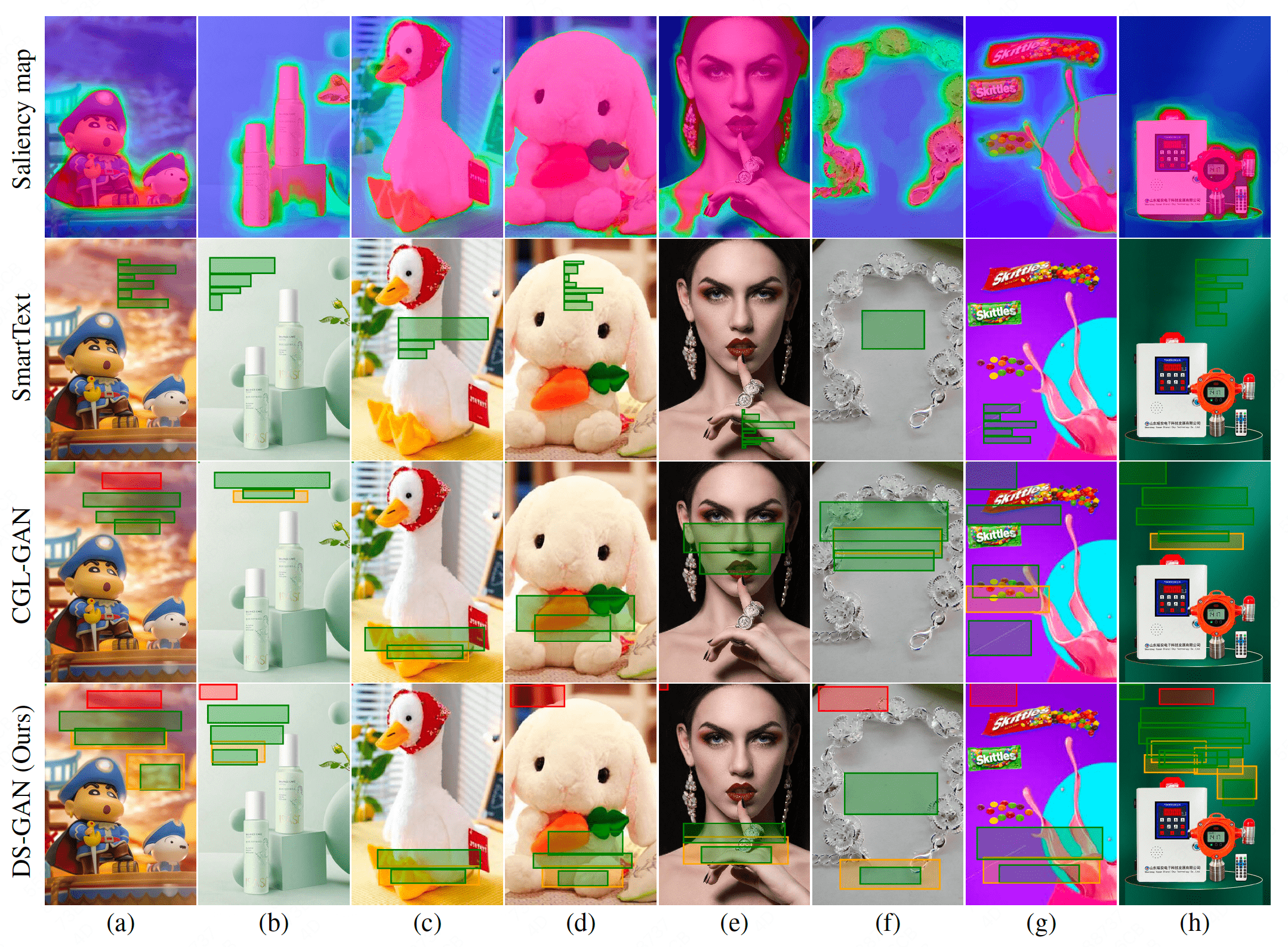
Figure 3. Comparison of layouts generated by different approaches.
Add new results!
Please send your results and publication to 2401112164@stu.pku.edu.cn. We will update them on the leaderboard!
Source Code:
The source code has also been released on Github.
References:
-
[1] Gangwei Jiang, Shiyao Wang, Tiezheng Ge, Yuning Jiang, Ying Wei, and Defu Lian. Self-supervised text erasing with controllable image synthesis. In Proceedings of the ACM International Conference on Multimedia (ACM MM), page 1973–1983, 2022.
-
[2] https://unsplash.com/, https://www.freepik.com/, https://pixabay.com/, https://pngimg.com/, https://www.stickpng.com/
-
[3] Roman Suvorov, Elizaveta Logacheva, Anton Mashikhin, Anastasia Remizova, Arsenii Ashukha, Aleksei Silvestrov, Naejin Kong, Harshith Goka, Kiwoong Park, and Victor Lempitsky. Resolution-robust large mask inpainting with Fourier convolutions. In Proceedings of the IEEE/CVF Winter Conference on Applications of Computer Vision (WACV), pages 2149–2159, 2022.
-
[4] Bo Wang, Quan Chen, Min Zhou, Zhiqiang Zhang, Xiaogang Jin, and Kun Gai. Progressive feature polishing network for salient object detection. In Proceedings of the AAAI conference on artificial intelligence (AAAI), pages 12128–12135, 2020.
-
[5] Chenhui Li, Peiying Zhang, and Changbo Wang. Harmonious textual layout generation over natural images via deep aesthetics learning. IEEE Transactions on Multimedia (TMM), 2021.
-
[6] Min Zhou, Chenchen Xu, Ye Ma, Tiezheng Ge, Yuning Jiang, and Weiwei Xu. Composition-aware graphic layout GAN for visual-textual presentation designs. In Proceedings of the International Joint Conference on Artificial Intelligence (IJCAI), pages 4995–5001, 2022.
-
[7] HsiaoYuan Hsu, Xiangteng He, Yuxin Peng, Hao Kong and Qing Zhang, “PosterLayout: A New Benchmark and Approach for Content-Aware Visual-Textual Presentation Layout”, 36th IEEE Conference on Computer Vision and Pattern Recognition (CVPR), Vancouver, Canada, Jun. 18-22, 2023.
Contact:
Questions and comments can be sent to 2401112164@stu.pku.edu.cn.